StemGNN
- What problem is StemGNN solving:
- intra-series temporal pattern: DFT
- Each series
- inter-series correlations
- At each step, the interactions between nodes
- reversible operator
- Example problem
- Covid cases: DE, AT, NL, …
- Predicting each country without considering the interactions between them
- Or introduce the people flow between them
- intra-series temporal pattern: DFT
- GFT:
- Completes DFT as it takes care of the inter-series correlations
- one extra slide for this topic
- Convolutions on Graphs
- one extra slide for this topic
Graph Basics
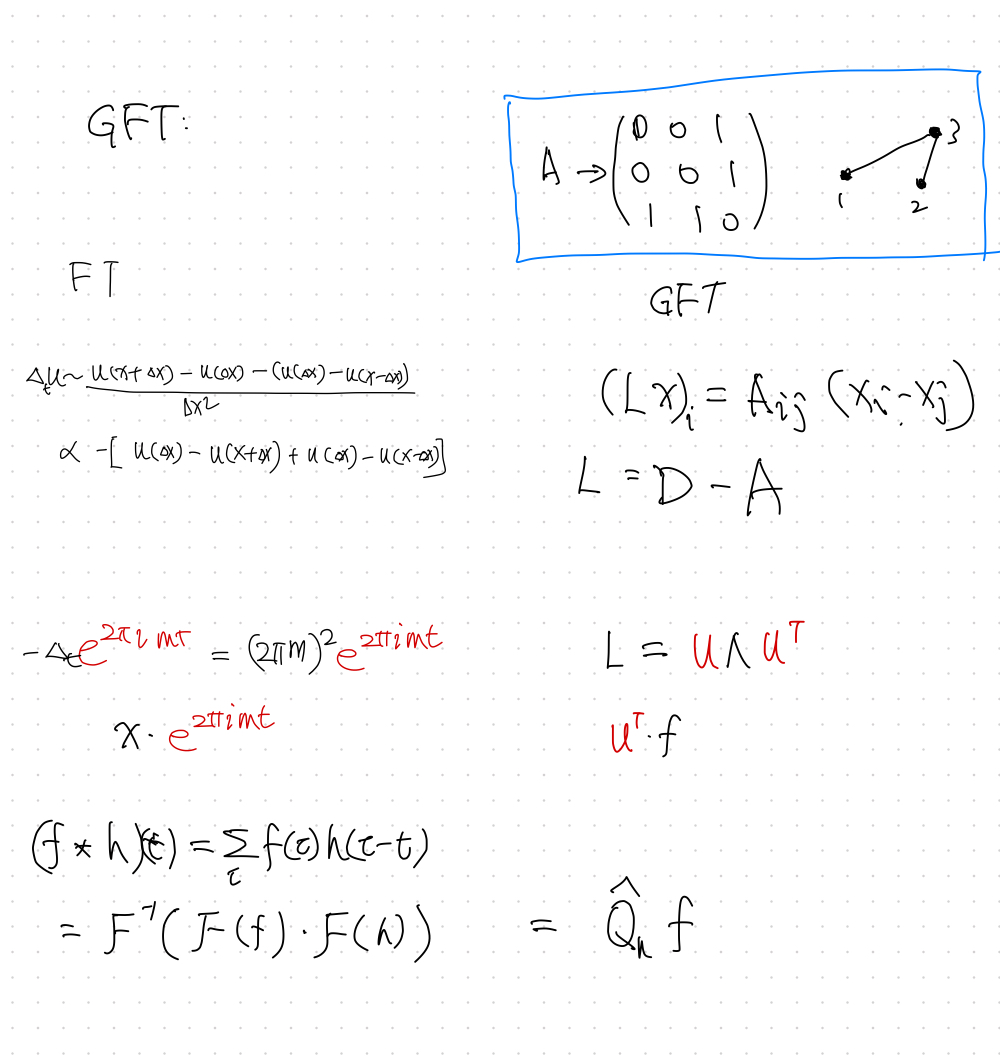
How to build the graph
- “self-attention”:
- outer product of key, query, as the adjacency matrix
- key, query are of length # of ndoes
- outer product of key, query, as the adjacency matrix
Weights and Biases
- LC
- 1DConv
- GLU
- FC
Experiments
- Traffic adjacency matrix
- neighbouring sensors have higher correlations
- Covid
- Neighbouring countries have higher correlation
- Spetral analysis:
- Some eigenvectors have clear meanings
Week spots
- Paper and code are not consistent
- Static graph
Architecture
stateDiagram-v2
with_spectral_matrix_representation: Spectral Matrix Representation
with_temporal_patterns_in_freq_domain: Temporal Patterns in Frequency Domain
with_latent_correlation: Latent Correlation Representation
dft_repr: Discrete Fourier Transformed Representation
conv_dft_repr: Convolved DFT Representation
[*] --> with_latent_correlation: Latent Correlation Layer
with_latent_correlation --> with_latent_correlation
state with_latent_correlation {
state with_spectral_matrix_representation {
[*] --> dft_repr: Discrete Fourier Transform
dft_repr --> conv_dft_repr: 1DConv
conv_dft_repr --> gated_conv_dft_repr: Gated Linear Unit
gated_conv_dft_repr --> inverse_dft_gated_conv_dft_repr: IDFT
}
[*] --> with_spectral_matrix_representation: Graph Fourier Transform
note right of with_spectral_matrix_representation: This happens in Spe-Seq Cell
with_spectral_matrix_representation --> with_temporal_patterns_in_freq_domain
with_temporal_patterns_in_freq_domain --> with_conv_temporal_patterns: Graph Convolution
with_conv_temporal_patterns --> with_timeseries_restored: IGFT
with_timeseries_restored --> Forecast
with_timeseries_restored --> Backcast
}
note right of with_latent_correlation: This is done in StemGNN Block
with_latent_correlation --> [*]
Planted:
by L Ma;
Similar Articles:
Lei Ma (2022). 'StemGNN', Datumorphism, 01 April. Available at: https://datumorphism.leima.is/reading/stemgnn/.