Continuous Ranked Probability Score - CRPS
The Continuous Ranked Probability Score, known as CRPS, is a score to measure how a proposed distribution approximates the data, without knowledge about the true distributions of the data.
Definition
CRPS is defined as1
$$ CRPS(P, x_a) = \int_{-\infty}^\infty \lVert P(x) - H(x - x_a) \rVert_2 dx, $$
where
- $x_a$ is the true value of $x$,
- P(x) is our proposed cumulative distribution for $x$,
- $H(x)$ is the Heaviside step function
$$ H(x) = \begin{cases} 1, &\qquad x=0\\ 0, &\qquad x\leq 0\\ \end{cases} $$
- $\lVert \cdot \rVert_2$ is the L2 norm.
Explain it
The formula looks abstract on first sight, but it becomes crystal clear once we understand it.
Note that the distributions that corresponds to a Heaviside CDF is the delta function $\delta(x-x_a)$. What this score is calculating is the difference between our distribution and a delta function. If we have a model that minimizes CRPS, then we are looking for a distribution that is close to the delta function $\delta(x-x_a)$. In other words, we want our distribution to be large around $x_a$.
To illustrate what the integrand $\lVert P(x) - H(x - x_a) \rVert_2$ means, we consider several scenarios.
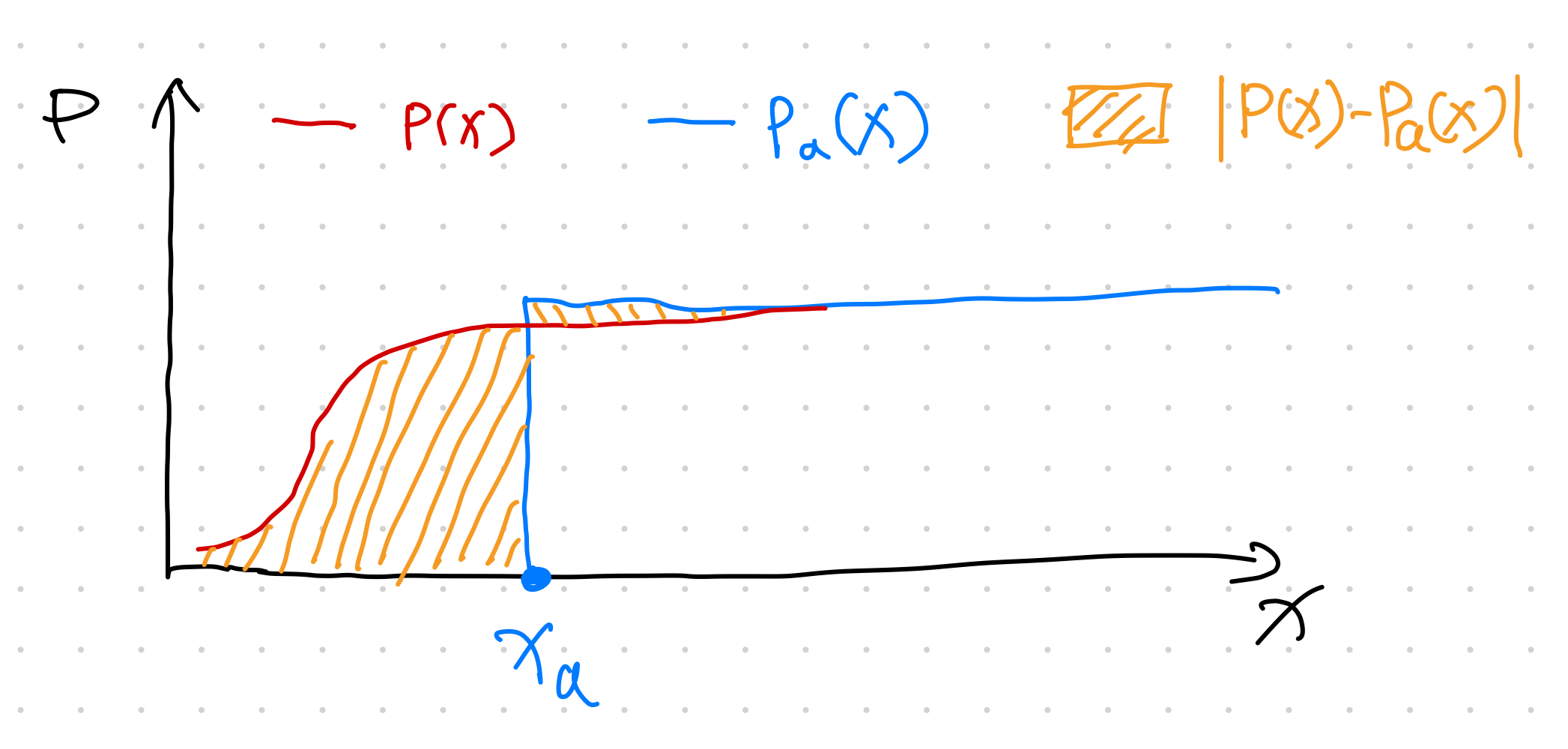
When the proposed CDF $P(x)$ is reaching 1 faster
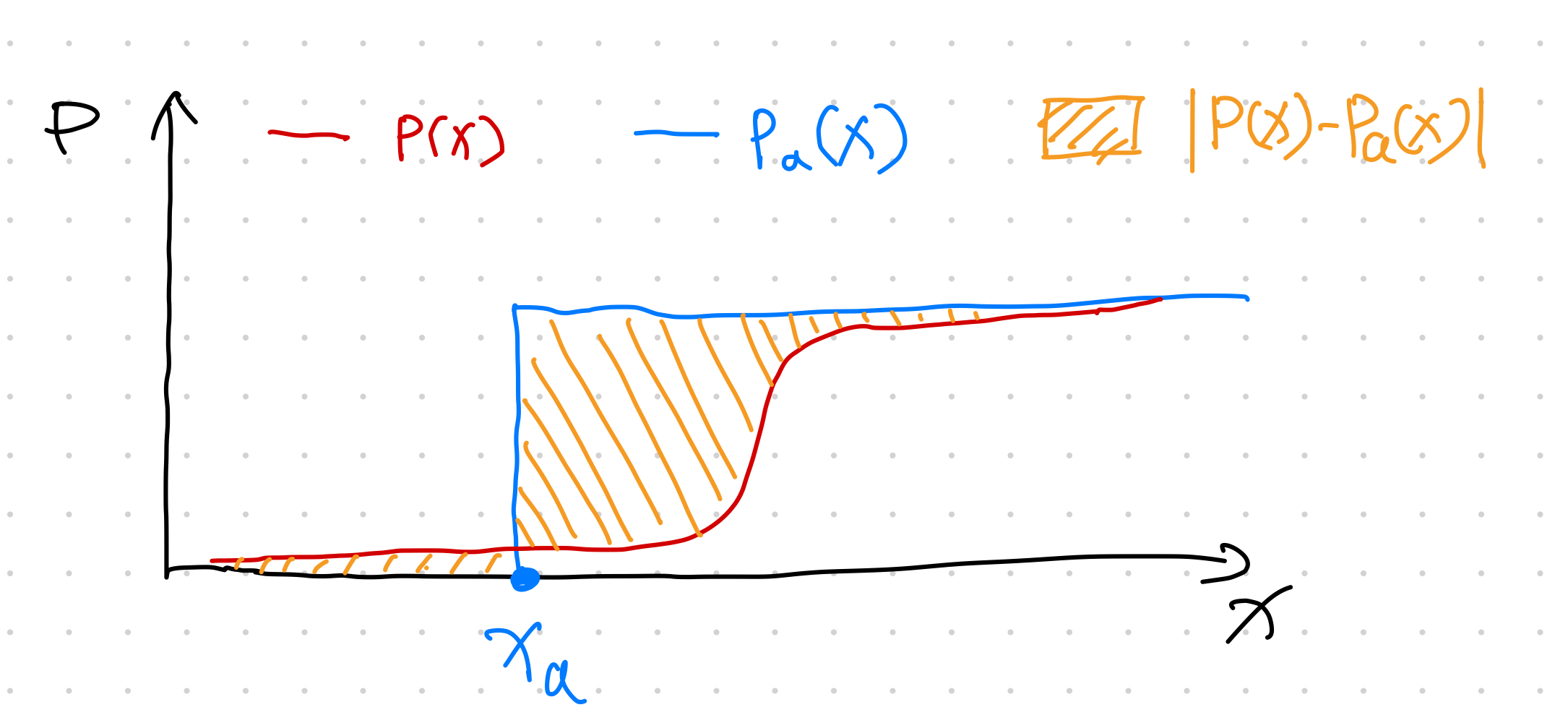
When the proposed CDF $P(x)$ is reaching 1 slower
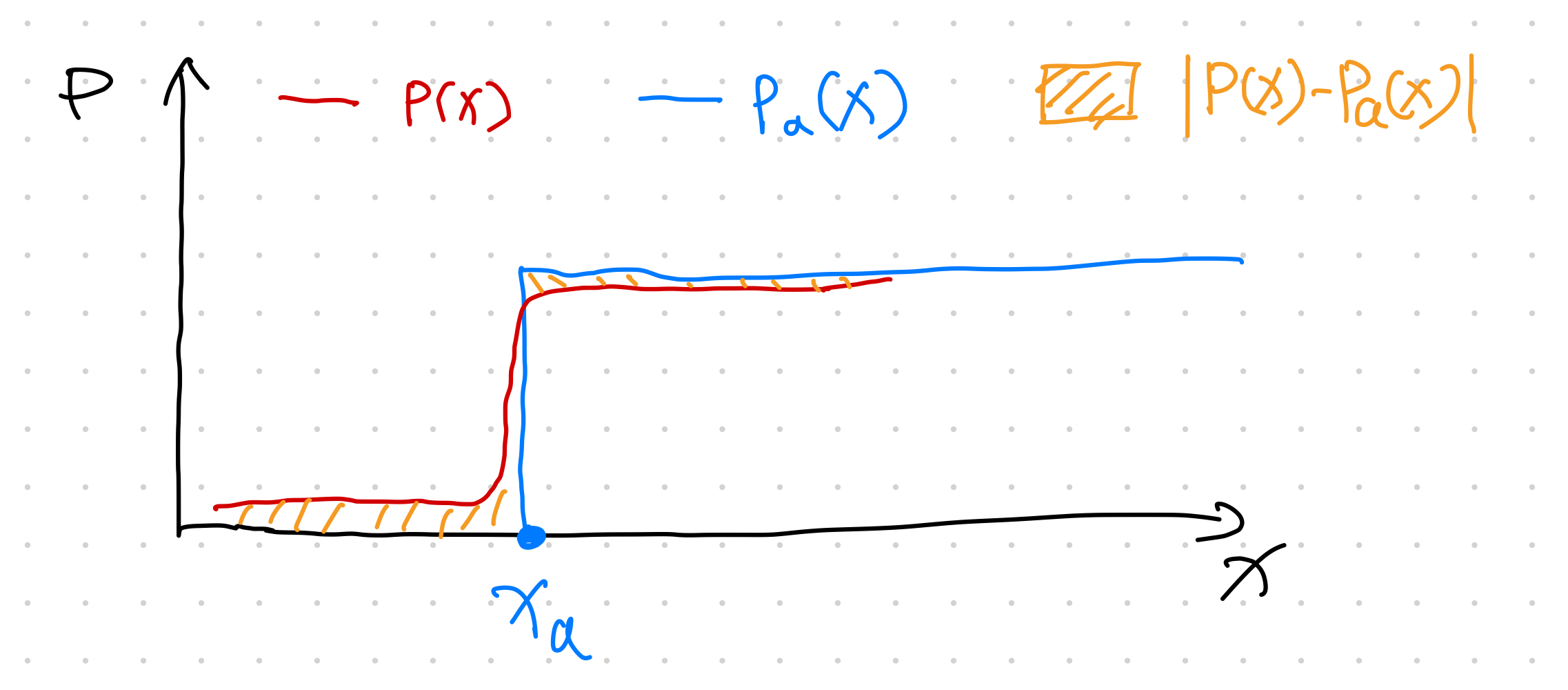
When the proposed CDF $P(x)$ is close to the Heaviside function
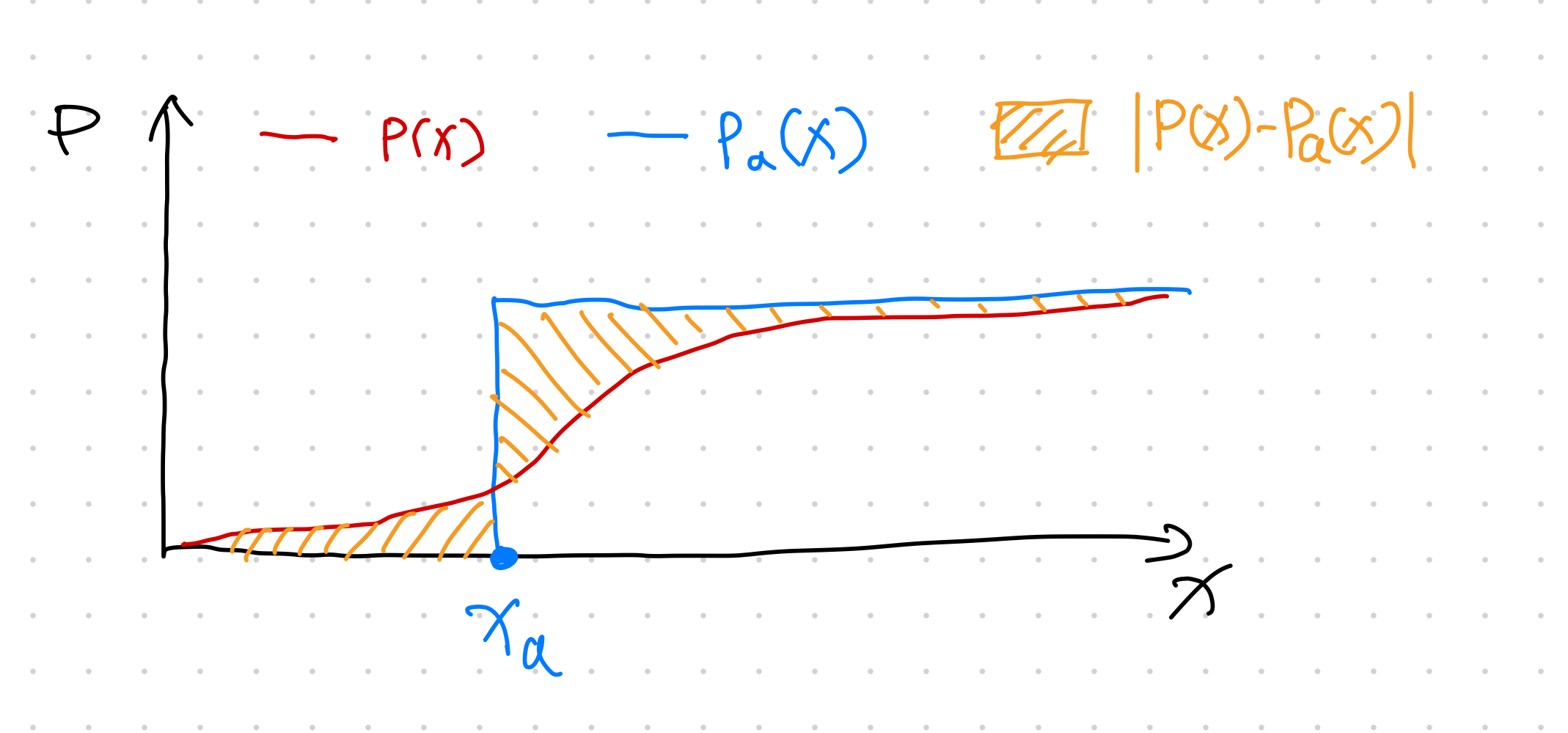
When the proposed CDF $P(x)$ is dispersed around $x_a$
The shade areas determines the integrand of the integral in CRPS. The only way to get a small score is to choose a distribution that is focused around $x_a$.
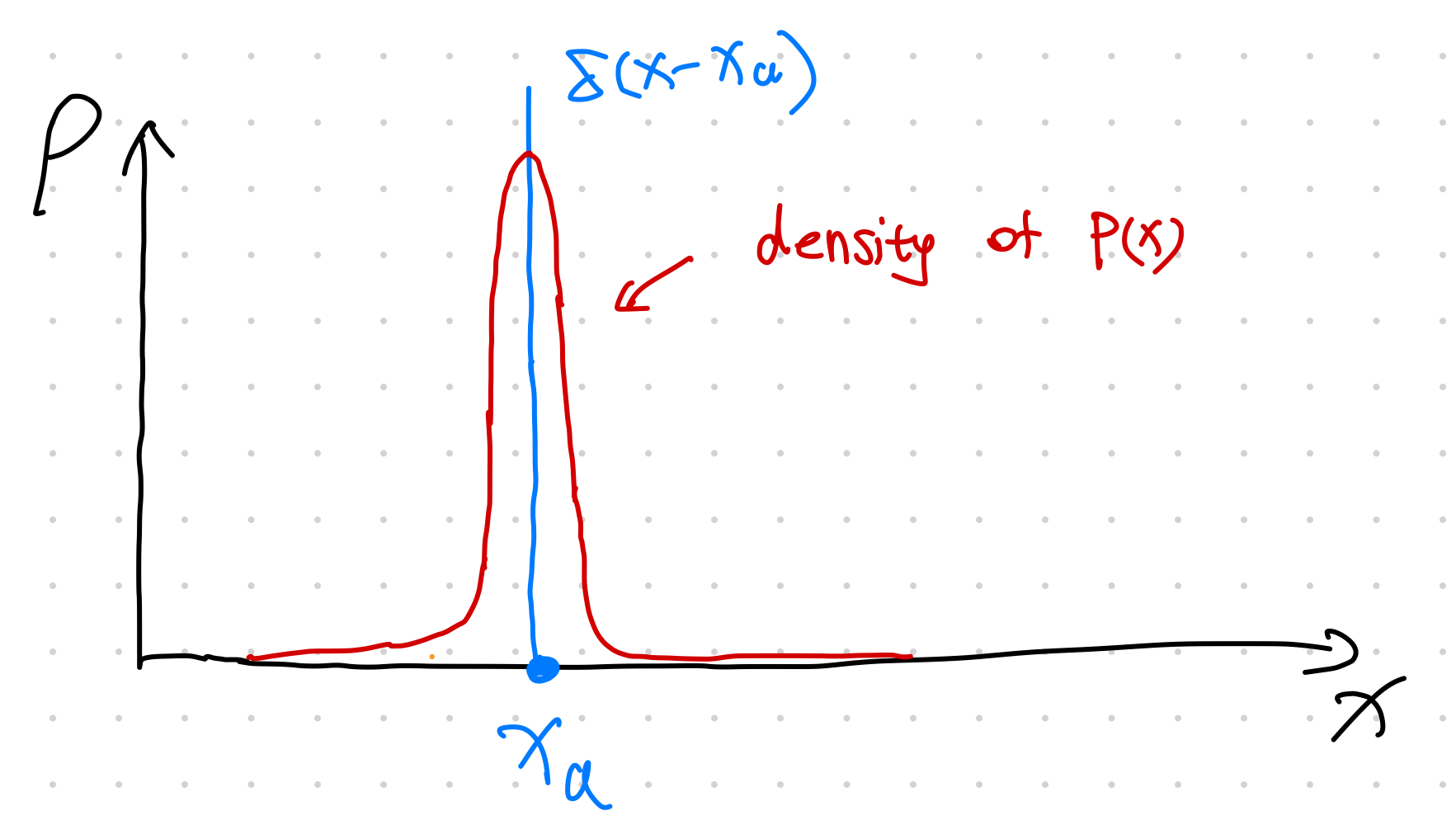
densities of $P(x)$ and $H(x-x_a)$
Compared to f-Divergence
Compared to [[KL Divergence]] KL Divergence Kullback–Leibler divergence indicates the differences between two distributions or more generally [[f-Divergence]] f-Divergence The f-divergence is defined as1 $$ \operatorname{D}_f = \int f\left(\frac{p}{q}\right) q\mathrm d\mu, $$ where $p$ and $q$ are two densities and $\mu$ is a reference distribution. Requirements on the generating function The generating function $f$ is required to be convex, and $f(1) =0$. For $f(x) = x \log x$ with $x=p/q$, f-divergence is reduced to the KL divergence $$ \begin{align} &\int f\left(\frac{p}{q}\right) q\mathrm d\mu \\ =& \int \frac{p}{q} \log \left( \frac{p}{q} \right) \mathrm d\mu … , CRPS is comparing our proposed CDF to the Heaviside CDF.
Compared to Likelihood
Gebetsberger et al found that CRPS is more robust but produces similar results if we have found a good assumption about the data distribution2.
Applications
One quite interesting application of the CRPS is to write down the loss for an the quantile function3.
Hersbach2000 Hersbach H. Decomposition of the Continuous Ranked Probability Score for Ensemble Prediction Systems. Weather Forecast. 2000;15: 559–570. doi:10.1175/1520-0434(2000)015<0559:DOTCRP>2.0.CO;2 ↩︎
Gebetsberger2018 Gebetsberger M, Messner JW, Mayr GJ, Zeileis A. Estimation Methods for Nonhomogeneous Regression Models: Minimum Continuous Ranked Probability Score versus Maximum Likelihood. Mon Weather Rev. 2018;146: 4323–4338. doi:10.1175/MWR-D-17-0364.1 ↩︎
Gouttes2021 Gouttes A, Rasul K, Koren M, Stephan J, Naghibi T. Probabilistic Time Series Forecasting with Implicit Quantile Networks. arXiv [cs.LG]. 2021. doi:10.1109/icdmw.2017.19 ↩︎
- Hersbach2000 Hersbach H. Decomposition of the Continuous Ranked Probability Score for Ensemble Prediction Systems. Weather Forecast. 2000;15: 559–570. doi:10.1175/1520-0434(2000)015<0559:DOTCRP>2.0.CO;2
- Gouttes2021 Gouttes A, Rasul K, Koren M, Stephan J, Naghibi T. Probabilistic Time Series Forecasting with Implicit Quantile Networks. arXiv [cs.LG]. 2021. doi:10.1109/icdmw.2017.19
- Gebetsberger2018 Gebetsberger M, Messner JW, Mayr GJ, Zeileis A. Estimation Methods for Nonhomogeneous Regression Models: Minimum Continuous Ranked Probability Score versus Maximum Likelihood. Mon Weather Rev. 2018;146: 4323–4338. doi:10.1175/MWR-D-17-0364.1
L Ma (2022). 'Continuous Ranked Probability Score - CRPS', Datumorphism, 03 April. Available at: https://datumorphism.leima.is/cards/time-series/crps/.